Putting an AI turbo in land surface models
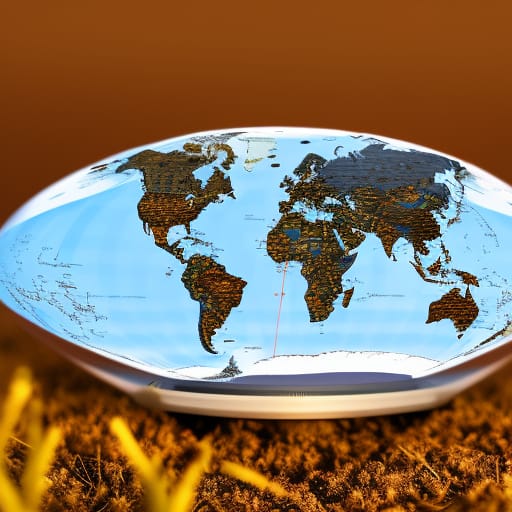
The rapid advancement of the big-data-big-model has reached its bottleneck: high computational requirements prevent further development of Terrestrial Biosphere Models (TBM) that need to be integrated over long time scales to simulate the distribution of ecosystems carbon and nutrient pools and fluxes.
To tackle this grand challenge, we developed a machine-learning acceleration (MLA) tool for the most resource-consuming step in TBMs: the equilibration of biogeochemical cycles (spin-up). We show that an unoptimized MLA reduced the computation demand by roughly 80% for global studies via interpolating the equilibrated state of biogeochemical variables for a subset of model pixels.
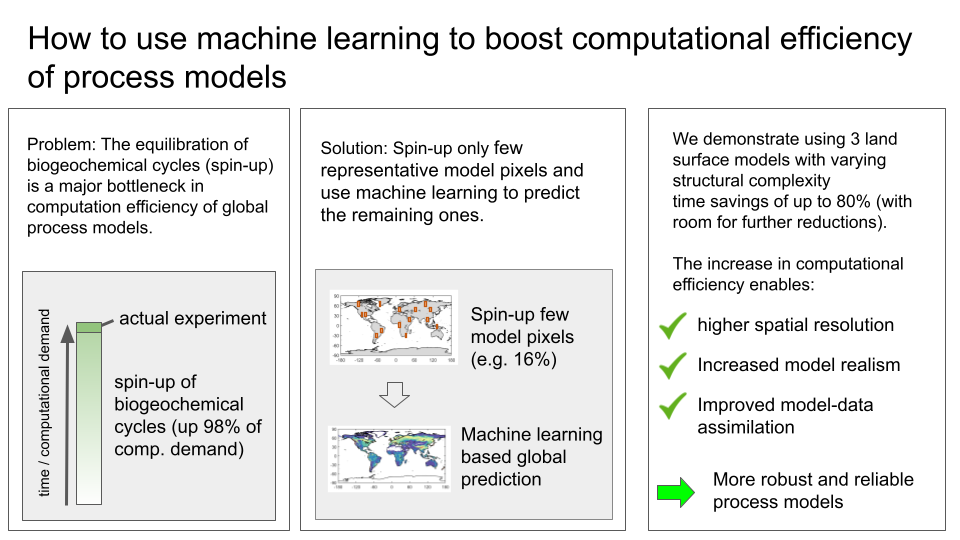
Encouraged by the large computational time saving of a first pragmatic implementation of the concept by Yan Sun combining unsupervised and supervised machine learning, we are developing an open software tool for land surface models. We believe time savings of one order of magnitude are in reach which would open up pathways to increase the realism and robustness of conventional land surface models.
Open-access article: Sun, Y., Goll, D. S., Huang, Y., Ciais, P., Wang, Y.-P., Bastrikov, V., & Wang, Y. (2023). Machine learning for accelerating process-based computation of land biogeochemical cycles. Global Change Biology, 00, 1-14. https://doi.org/10.1111/gcb.16623
Open source software for use with ORCHIDEE family models SPINacc